What is data quality and why is it important for business Success?
Data quality is the measure of how accurate, complete, valid, and relevant the data your business uses is. We’re sharing best practices for business success.
Published:
Last updated:
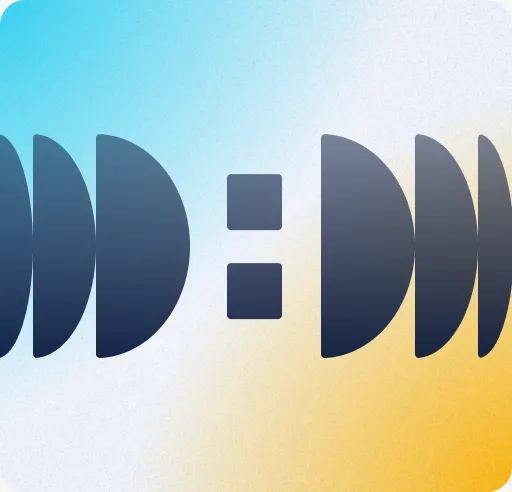
Finding it hard to keep up with this fast-paced industry?
Data quality: How to create it and why it matters
If an organization has high-quality data, it can make better strategic decisions. It’s that simple. Here’s why data quality matters in business and how your company can create the best version of the data it uses.
What is data quality?
Data quality is the measure of how accurate, complete, consistent, valid, and relevant the data your business uses is.
It certifies that the information used for activities like analysis, reporting, and decision-making is reliable and fit for purpose, so it's an important part of the data management process.
The higher the quality of data you have at your disposal, the better you'll be able to use it. So by regularly monitoring your data quality, your organization can quickly identify and address potential issues that could impact your operation.
Most notably, this applies to customer service, employee productivity, and strategic initiatives.
Data quality vs data integrity: What’s the difference?
While they are closely related, data quality and data integrity serve entirely different purposes.
Data quality measures how accurate, complete, consistent, and valid data is for business use. It focuses on whether the information meets expectations and supports effective decision-making.
The main purpose of data integrity is to make sure that data remains accurate, unchanged, and reliable throughout its lifecycle. Effectively, this consistency is carried from the point of its creation to the point where it's stored and shared.
With strong data integrity in place, businesses can protect themselves against unauthorized changes. At the same time, creating high data quality ensures it remains useful to them in the future.
Together, they form the foundation of trusted data, which facilitates better governance, compliance, and business outcomes.
6 key characteristics that define data quality
If you want to build a trusted data system, it's essential to understand the main characteristics of data quality. Overall, there are six aspects you should be aware of:
- Accuracy: Data should be free from errors and correctly reflect the real-world event, object, or concept it represents.
- Completeness: It must include all the required fields and values that are needed for its intended purpose, without omitting important details.
- Consistency: All data sets should remain uniform across different databases and systems. In doing this, you’ll reduce the potential for conflicts and contradictions.
- Validity: Data must comply with defined formats, rules, and acceptable range, which helps ensure inputs are structured correctly.
- Timeliness: Your data should be available on demand and regularly updated. This will enable your company to make effective, real-time decisions.
- Uniqueness: Each data entry should be distinct, with no duplicates or redundant records. If they aren't, it could skew your insights and predictions.
Overall, the more an organization can recognize these dimensions, the more it will help them to assess, measure, and improve their levels of data quality.
Why high-quality data matters for your business
High-quality data is one of the most valuable assets any business can have. It can support strategic initiatives, drive operational efficiency, and strengthen trust across the organization.
However, it can only do this if it meets the right standards. Here are some compelling reasons why you should invest in data quality:
Reliable decision-making
Quality data provides a trustworthy foundation for insights, which helps business leaders to make more confident and informed choices.
Operational efficiency
Businesses that work with consistent, validated data don't often suffer from bottlenecks. This can speed up workflows and minimize the cost of redundant work across systems.
Superior customer experiences
Accurate customer, product, and address data helps companies provide more personalized and relevant interactions with their customers. This improves how they deliver their service and often results in them being more loyal.
Cost reduction
The quicker you can identify and fix data errors, the fewer resources you'll waste. Subsequently, your profitability will increase, and you will experience minimal operational disruptions.
Regulatory compliance
When businesses produce strong data quality, their records are more likely to be complete, valid, and consistently maintained. As a result, they're better equipped to meet their compliance obligations.
High-quality data can support every aspect of an organization. Without it, even the best strategies, systems, and teams have the potential to falter.
How data quality impacts businesses
The true value of data quality becomes clear when you see how it affects real-world operations. See how these leading companies have seen data quality impact their outcomes with these examples that illustrate how data quality drives (or damages) business success.
Reliable decision-making: American Express
American Express relies on clean, validated data to detect fraud and segment its customers. Their ability to use trusted data helps them offer personalized products and identify deceitful activity within seconds.
Without high-quality data, the speed and accuracy of their decision-making may suffer. This could potentially impact customer trust and their overall operational security.
Operational efficiency: Mayo Clinic
The Mayo Clinic faced challenges with inconsistent patient records across departments, which affected their treatment plans and billing.
However, by building the Patient Misidentification Notification System (PMNS), they were able to automatically notify over 400 internal systems when patients' records have to be merged or unmerged.
This helped them improve their operational efficiency and delivery of patient care. They were also able to significantly slash the number of duplicate records they had on their databases.
Superior customer experiences: Amazon
Amazon enjoys an industry-leading customer experience, which is fueled by its data quality.
It revolves around the collation of accurate product information, real-time inventory updates, and precise customer addresses. The result of this is a seamless transaction with fast deliveries and personalized recommendations.
Regulatory compliance: Pfizer
Pfizer has been named one of the world’s most ethical companies. One of the main reasons why is that it maintains impeccable data accuracy to help meet strict pharmaceutical regulatory compliance.
During drug development and clinical trials, validated and consistent data is collated to ensure that product safety reporting and regulatory submissions are accurate. This enables them to avoid delays and penalties that may negatively impact their operation.
5 ways to improve data quality
If you want to improve the quality of your company’s data, best practice is to employ a proactive and strategic approach.
Here are 5 pro strategies you can adopt to strengthen your data quality initiatives:
Data quality assessment
It's a good idea to regularly assess your data quality by using defined metrics such as accuracy, completeness, and consistency.
The more you measure and monitor your data, the easier it will be to pinpoint issues early, as opposed to reacting to errors that may negatively impact your business outcomes.
Data cleansing and standardization
Cleansing data is an important step in maintaining data quality. By removing duplicates and correcting errors, you will create formats that share a common standard.
If you don’t achieve such standardization, you run the risk of even clean data causing inconsistencies across your systems.
Data profiling
Before integrating new data into your core systems, take the opportunity to analyze data patterns and identify anomalies.
Skipping these profiling steps puts you at greater risk of inviting poor data quality issues that could compromise the integrity of your data lifecycle.
Automation and AI in data quality
Automation tools and AI-based solutions are ideally suited to maintaining good data quality.
They can be used for tasks like handling repetitive quality checks, real-time validations, and data enrichment. Manual processes often introduce errors and are hard to scale, so these tools can minimize those risks.
Building a data quality culture
Poor data often stems from a lack of ownership. Therefore, it's wise to develop a strong culture that encourages everyone to play a part in maintaining your trusted data.
Training your employees, empowering data stewards, and encouraging a culture of accountability are effective approaches to achieving this.
Common data quality challenges and how to solve them
Even with the best planning, it can be a challenge to maintain high-quality data. Businesses can face a range of obstacles that they will need to counter.
Here are some of the most common challenges and how you can overcome them:
Data volume and velocity
Traditional systems are not well-equipped to handle the massive scale and speed of modern big data and real-time streams – they can be easily overwhelmed by them.
To counter this, you should adopt scalable cloud-based platforms and real-time data streaming tools like Apache Kafka, which are far more capable of efficiently managing data pipelines,
Data silos and integration
If data is stored in isolated systems, it can create inconsistencies that may reduce the integrity of your data value. Thankfully, there are several integration platforms and master data management (MDM) solutions you can use to unify datasets. Adopting them will help to create a single source of truth across your enterprise.
Emerging technologies
Emerging technologies like AI, machine learning, cloud computing, and edge systems are bringing new opportunities for companies to strengthen their data quality.
By investing in data quality management tools, your organization will be better set up to handle hybrid environments. As well, if you prioritize platforms that offer built-in governance and validation features, you’ll enjoy better compliance as well.
Data governance
In terms of data governance, inconsistent practices occur when there is a lack of clear policies, roles, and ownership.
It's important for any company to establish a formal data governance framework, to define standards, assign data stewardship roles, and enforce data quality rules across the organization.
Data ethics and bias
AI models and datasets can succumb to bias if they are unchecked, which can lead to unfair outcomes. So it's vital to build ethical review processes into data projects. You can also use bias detection tools to shore up the results.
At the same time, maintaining transparency in how data is collected, labeled, and used is also a good way to achieve authenticity.
Summing up
Good data quality is an essential part of modern business. By implementing data quality solutions and improving your data accuracy, businesses can make better business decisions and secure long-term success.
Ready to take control of your data quality?
Discover how RecordPoint’s intelligent data management platform can help you establish trust, meet compliance requirements, and unlock tangible business value. Contact us today to get started.
FAQs
What are the key data quality dimensions?
The main dimensions for data quality are accuracy, completeness, consistency, validity, timeliness, and uniqueness. They help to measure how trustworthy and useful your data is.
How is data quality measured?
Data quality is assessed through metrics like error rates, completeness scores, consistency checks, and adherence to business rules. Regular data profiling and monitoring also help businesses maintain quality data over time.
What causes poor data quality?
Poor data quality often comes from human error, inconsistent data entry, siloed systems, or outdated information. In addition, a lack of governance and unclear data ownership can make the problem worse.
Can data quality impact compliance?
Yes. Companies found to have inaccurate or incomplete data can be deemed to have compliance failures. This can lead to fines and substantial damage to their reputation. That's why it is imperative for businesses to have strong data governance and quality control to meet regulatory standards.
What is the role of data stewards?
The primary role of a data steward is to manage and enforce data quality standards. They ensure that data remains accurate, consistent, and properly governed across its entire lifecycle.
Discover Connectors
View our expanded range of available Connectors, including popular SaaS platforms, such as Salesforce, Workday, Zendesk, SAP, and many more.