Data discovery vs business intelligence: Are they the same?
Data is a valuable asset for almost every organization. It offers a competitive advantage, drives every big idea, and informs every critical decision. But as the volume of data the world generates grows year on year, how can businesses keep pace and leverage these modern data assets for their own benefits?
Published:
Last updated:
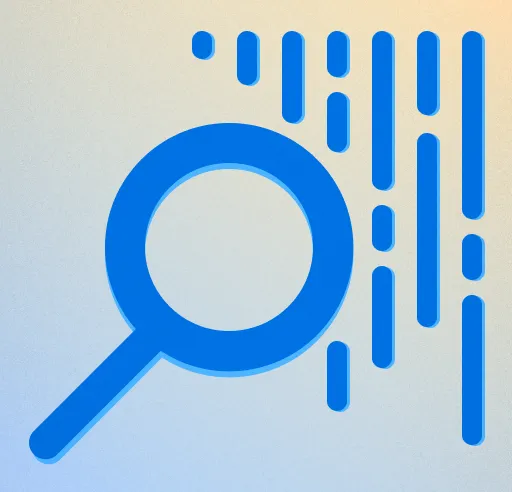
Finding it hard to keep up with this fast-paced industry?
What is business intelligence?
Business intelligence (BI) encompasses the methods and tools businesses use to convert historical data into insights they can use to make everyday decisions. As such, BI involves a range of methodologies, including:
- Data collection: Collating data from various sources
- Data curation: Organizing this collected data into a centralized data system
- Querying: Finding answers to specific questions using the curated data
- Reporting: Collating these insights in an accessible report
- Visualization: Visualizing data, often supported by tools such as Power BI
- Dashboard development: This is more common with modern BI, and involves building dashboards to monitor essential metrics in real-time.
This list isn’t exhaustive. However, it exemplifies how many different elements contribute to business intelligence. It is a holistic strategy to help businesses make informed decisions based on past data.
What’s the process?
How does this work in practice? A typical scenario might look like this.
- Say a business wants to analyze its lead generation from a recent marketing campaign.
- An expert collates the data from the organization’s website and CRM solutions.
- The expert completes the curation process.
- They store the curated data in the organization’s enterprise data warehouse.
- They then use the data to create a standardized, accessible report.
- Lastly, they present this report so that management can interpret the results.
This is just one of many use cases for BI. We’ll discuss more later. But for now, let’s take a look at data discovery.
What is data discovery?
Data discovery is the process of classifying and exploring siloed data stores to detect patterns and uncover insights. Think of it as arranging a chaotic mess of tiles into a mosaic.
Businesses routinely battle with disparate data siloed across databases, data warehouses, and external sources like social media sites. Data discovery helps analysts collate this data and then use it to discover actionable insights that may not be immediately obvious.
They can even do this proactively to identify and address concerning trends before they become a problem.
What’s the process?
How might data discovery work in practice? Here’s a common 5-step process.
- Objectives: The business loosely defines the objectives of data discovery, whether that be to explore a problem or collect data for compliance with regulations.
- Mapping: A team member uses a data discovery tool to map out structured and unstructured data sources and hones in on the most relevant data. This also includes mapping data flows and data lineage.
- Classifying: The analyst profiles, tags, and classifies the data, standardizing the information and removing anomalies. They will ensure the data is formatted properly so it is easy to read to make data discoverability easier. This section also encompasses metadata management.
- Exploration: With standardized data, the analyst begins exploring the data sets to uncover insights.
- Recording: The analyst records or reports their findings. This can be fed into BI to create visual reports or back into data discovery tools to allow for further investigation.
Many of the workflows in steps two, three, and even four can be automated today with AI applications. This takes a lot of the tedious, difficult work out of data discovery, meaning even those without IT skills can begin discovering insights from the data at hand.
Business intelligence vs data discovery
Data discovery and business intelligence are both about attempting to use data assets to help businesses make improved, data-led decisions. But there are some differentiators.
1. Purpose
BI - Purpose
BI usually aims to answer predefined questions. For example, a business leader may want to answer a query like, ‘Are we meeting our KPI for customer retention this year?’ They will then ask an expert to draw historical information from the organization’s data warehouse.
The expert will access the organization’s internal warehouse to gather the information they need. They will then present this information in a standardized report so that the organization's management can determine whether the company has met its business outcomes.
Data discovery - Purpose
Data discovery is much more free-form. It’s about exploring data sets to uncover insights that may not be obvious.
The analyst dives into the collated data set with fewer preconceptions about what they hope to find. The goal is to explore the data and find trends and patterns—not necessarily to answer a specific query. This approach helps businesses discover risks and opportunities proactively. In contrast, BI is more prescriptive and based on historical data.
2. Data sources
BI - Data sources
Business intelligence usually leverages structured data sources stored in databases (like spreadsheets) and data warehouses (central data repositories). This data is collected and stored in a standardized format to ensure data quality and accessibility, making it simpler for analysts to generate consistent reports.
Data discovery - Data sources
While data discovery can and does involve structured stores, it can also analyze a broader spectrum of custom data types, such as semi-structured and unstructured on-premises and cloud data. It can incorporate multimedia files, social media content, and external data sources like public databases and industry reports.
And, crucially, it doesn’t require a centralized data warehouse. Many tools can classify and organize data in situ.
3. Complexity
BI - Complexity
Navigating BI tools requires a specific skill set, as does interpreting complex records. These tools also typically take months to create because of the need for enterprise data. The reports are typically broader and more prescriptive, providing high-level overviews supported by metrics. As such, BI is utilized by expert data scientists, such as data engineers and analysts.
Data discovery - Complexity
Data discovery tools are easy to use and offer guided advanced analytics. Many leverage artificial intelligence and automatically categorize and standardize raw data. This opens the door for all business users to utilize data discovery in their workflows, even those without IT expertise.
For instance, a marketing team could collate and explore social media data to decide which content types produce the best results.
4. Scope
BI - Scope
BI still encompasses data exploration, but the scope of analysis is more limited. Analysts usually already know which datasets and metrics to extract before they begin to interact with the information. That’s a benefit when selecting financial metrics to answer specific questions but a drawback when there might be multiple versions of the truth.
Data discovery - Scope
Data discovery is much more open. It takes an exploratory approach rather than relying on predefined data to make evidence-based decisions. If someone discovers a unique trend, they can pursue the lead dynamically to find out more.
This is largely because data discovery isn't tied to the organization's data warehouse, meaning users have more freedom to discover hypotheses and find creative solutions.
5. Reporting
BI - Reporting
Business intelligence utilizes standardized report templates. These reports are designed to be repeatable and easily quantifiable, such as when tracking quarterly sales figures against sales forecasts. Data can then be handpicked from the organization’s data catalog to support this theory.
Data discovery - Reporting
With data discovery, reports aren't always considered the end goal. Instead, the goal is more open-ended and down to the discovered data. Don't get us wrong. Insights from data discovery are often still visualized to make them easier to understand. But this isn't an essential part of the data discovery strategy—more an optional extra.
Data discovery and business intelligence are intertwined
It’s worth noting that data discovery and business intelligence, while different, aren’t mutually exclusive.
Going back to the ‘sales figures versus sales forecasts’ BI report, for example. If the company determined there was a disparity between the expected sales and the actual sales, they may use data discovery to determine why this is the case.
Once they’ve explored the data sets to glean insights, they can then use BI once again to collate the findings in a visual report. If the report raises more questions and hypotheses, the data discovery process can begin again.
You can see how the two methods can link together in a feedback loop to provide ongoing insights and data visualizations to business data leaders. We suggest using both. That said, each strategy has its ideal use cases. Let's explore some of those now.
When to use business intelligence versus data discovery
Data discovery and business intelligence each serve different purposes. It isn’t a ‘one or the other’ situation, but we recommend selecting your method based on the task at hand. Let’s look at common use cases for each.
Business intelligence use cases
BI works best when it comes to tracking a specific metric or answering a question based on readily available data. It is more prescriptive and single-minded than data discovery.
This isn’t necessarily a bad thing, though. In fact, BI is better than data discovery for several use cases.
- Monitoring trends over time: BI is ideal for tracking standardized metrics, like units sold or average order value, over an extended period. Many BI tools allow for real-time monitoring, meaning reports and dashboards can be updated instantly to reflect new information.
- Inventory management: In a similar vein, BI tools are well-suited to monitoring stock levels and supply chain performance. All of this historical data is usually readily available in the organization’s data warehouse, so businesses can use BI to create accessible reports without requiring in-depth data discovery.
- Tracking KPIs: BI is essential for tracking key performance indicators. That could mean monitoring a marketing campaign or assessing financial metrics, for example. In short, if there’s readily-available historical data for analysis of a core metric, BI is a solid choice.
Data discovery use cases
Data discovery is an ongoing process. It does not necessarily require a motive. This open-ended problem-solving means data discovery has several excellent use cases. Let's detail a few now.
- Identifying the root cause of a problem. Data discovery is the ideal tool for finding solutions to an open-ended problem. It lets individuals analyze unstructured and structured sources to identify potential causes and create hypotheses for further investigation.
- Optimizing a solution. The cyclic nature of data discovery makes it ideally suited to ongoing optimization. This might be fine-tuning a marketing strategy or enhancing manufacturing, for instance. It opens the door for businesses to explore data creatively and develop unique optimization solutions.
- Maintaining data governance. The early stages of any data discovery strategy involve data preparation, as well as curating data and ensuring data quality for exploration. This allows users to track data lineage, ensure data observability, and maintain effective data management. As such, it contributes to better governance and easier compliance with data protection regulations.
Summing up
Data discovery and business intelligence are both essential aspects of an organization’s data strategy. BI is best for answering predefined questions and monitoring trends over time, while data discovery is suited to exploration. It uncovers insights and trends you otherwise may have missed.
However, both solutions stem from the same need—to drive better decision-making and create more opportunities for businesses to use their data to grow.
Our number one piece of advice? Use a platform to help you manage and automate data cleaning, data classification, and curation practice. When done manually, these processes take a long time. A platform will make these tedious jobs much easier.
For instance, using a platform with ML and AI capabilities will take much of the hassle out of data cataloging and classification. Aside from that, it’ll also help you comply with all of the rules and regulations surrounding data handling.
Above all, it’ll save you time. That’s time you can then spend discovering insights and drawing value from your data to make better decisions and grow your business.
The RecordPoint approach to data discovery
With RecordPoint, you can find and manage your data wherever it lies.
Our machine-learning model will automatically classify and standardize your structured and unstructured data sets. It will automatically remove any redundant, obsolete, or trivial datasets, leaving behind only the information you need to make better business decisions. You can then access your data directly from the platform without needing to catalog your information manually.
For business intelligence? Our automated data reporting feature will offer a comprehensive view of core metrics and organizational risk based on all of your data stores. With over 900 connection opportunities, you can even integrate our platform with your preferred BI solution, whether that be Power BI, Tableau, Qlik, or Looker.
Want to utilize data discovery? Our manage-in-place features let you search for data and explore where it lies rather than hunting through siloed stores. You’ll save all the hassle of manual exploration—giving you more time to analyze your data for valuable insights.
Why trust us? We’re the only SaaS records management platform to complete a formal third-party IRAP assessment. That means we’ve proven our commitment to data management, security, and compliance.
Want to learn more? Schedule a demo today and discover why we’re the trusted data solution for dozens of heavily regulated organizations.
Discover Connectors
View our expanded range of available Connectors, including popular SaaS platforms, such as Salesforce, Workday, Zendesk, SAP, and many more.